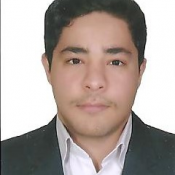
محمدرضا داودابادی
عنوان پایاننامه
پیشبینی مقدار شاخص های بازارهای مالی با استفاده از یک مدل مبتنی بر ترنسفورمر
- مقطع تحصیلی
- کارشناسی ارشد
- ساعت دفاع
- استاد راهنما
- فرزام متین فر
- دانشجو
- محمدرضا داودابادی
- استاد مشاور
- محمدرضا اصغری اسکوئی
- استاد داور
- نویده مدرسی