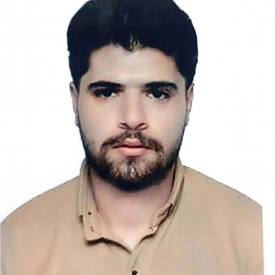
سالار بابازاده بهستانی
کاربرد شبکه های یادگیری عمیق آماری در قطعه بندی تصاویر پزشکی
- دانشجو
- سالار بابازاده بهستانی
- استاد راهنما
- وحید رضایی تبار
- استاد مشاور
- محمدرضا صالحی راد
- استاد داور
- فرزاد اسکندری
- مقطع تحصیلی
- کارشناسی ارشد
- تاریخ دفاع
- ۳۱ شهریور ۱۴۰۳
- ساعت دفاع
- چکیده
-
در این پایاننامه، به بررسی و بهبود روشهای قطعهبندی تصاویر سهبعدی MRI با استفاده از شبکههای عصبی بیزی و شبکه های خود رمزگذار متغیر پرداخته شده است. هدف اصلی این پژوهش، افزایش دقت و کارایی در تشخیص و تحلیل تصاویر پزشکی بهویژه در حوزه تومورهای مغزی است. با توجه به پیچیدگی و حجم بالای دادههای پزشکی، استفاده از روشهای پیشرفته یادگیری عمیق و شبکههای عصبی بیزی وخود رمزگذار متغیر میتواند به بهبود قابلتوجهی در فرایند قطعهبندی و تحلیل تصاویر منجر شود.
در این پژوهش، ابتدا به معرفی مبانی نظری مرتبط با تصاویر سهبعدی MRI و قطعه بندی تصاویر سه بعدی و شبکه های یادگیری عمیق آماری برای قطعهبندی این تصاویر پرداخته شده است. در ادامه، به پیش پردازش تصاویر سه بعدی و به طور ویژه به معرفی شبکههای عصبی بیزی و خود رمزگذار متغیر و کاربردهای آنها در قطعهبندی تصاویر پزشکی پرداخته شده است.
نتایج حاصل از این پژوهش نشان میدهد که استفاده از شبکههای عصبی خود رمزگذار متغیر میتواند دقت و اطمینان در تشخیص و تحلیل تصاویر پزشکی را نسبت به شبکه های عصبی پیچشی بیزی بهبود بخشد و همچنین خطاهای ناشی از نویز و تغییرات را کاهش دهد. که مقدار ضریب تاس برای شبکه ی عصبی خود رمزگذار متغیر برای سه ناحیه کل تومور، هسته تومور و ناحیه تومور به ترتیب برای داده های اعتبار سنجی ۰.۹۱ و ۰.۸۶ و ۰.۸۲ و برای داده های اعتبار سنجی ۰.۸۸ و ۰.۸۱ و ۰.۷۶ است.
در نهایت، پیشنهاداتی برای تحقیقات آتی و بهبود روشهای موجود ارائه شده است. این پژوهش میتواند به عنوان گامی مهم در جهت ارتقای کیفیت خدمات پزشکی و بهبود سلامت بیماران محسوب شود.
- Abstract
-
In this thesis, the ۳D MRI image segmentation methods have been investigated and improved using bayesian neural networks and variable self-encoder networks. The main goal of this research is to increase accuracy and efficiency in the diagnosis and analysis of medical images, especially in the field of brain tumors. Considering the complexity and high volume of medical data, the use of advanced deep learning methods and Bayesian neural networks and variable self-encoder can lead to a significant improvement in the image segmentation and analysis process.
In this research, firstly, theoretical foundations related to ۳D MRI images and segmentation of ۳D images and statistical deep learning networks for segmentation of these images have been introduced. In the following, the preprocessing of ۳D images and especially the introduction of Bayesian neural networks and variable self-encoder and their applications in medical image segmentation have been discussed.
The results of this research show that the use of variable self-encoding neural networks can improve accuracy and reliability in the diagnosis and analysis of medical images compared to Bayesian convolutional neural networks and also reduce errors caused by noise and changes. that the value of the Dice coefficient for the variable self-encoding neural network for the three tumor regions, tumor core and tumor region is ۰.۹۱, ۰.۸۶ and ۰.۸۲ for the validation data and ۰.۸۸, ۰.۸۱ and ۰.۷۶ for the validation data respectively.
Finally, suggestions for future research and improvement of existing methods are provided. This research can be considered as an important step in improving the quality of medical services and improving the health of patients.