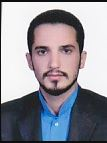
محمد اسدی
خوشه بندی داده های بالا بعد براساس آمیزه ای متناهی از توزیع های نرمال
- مقطع تحصیلی
- کارشناسی ارشد
- تاریخ دفاع
- ۲۸ شهریور ۱۴۰۲
- ساعت دفاع
- چکیده
-
هنگامی که خاصیت همگنی مشاهدات به راحتی در مِهدادهها و دادههای بالابّعد نقض میشود، گروهبندی دادهها در طول یک فرآیند یادگیری بدون نظارت بسیار مهم است. نسبت دادن برچسب یکسان به دادههایی که شباهت زیادی به یکدیگر و تفاوت بسیاری با سایر دادهها دارند یک فرآیند خوشهبندی است. یکی از کاربردیترین روشهای خوشهبندی زمانی است که از آمیزه متناهی توزیعهای آماری استفاده کنیم و رایجترین آنها آمیزه متناهی نرمال است. پیش از این عملکرد توزیع نرمال در خوشهبندی بررسی شده است اما استفاده از این توزیع زمانی که مشاهدات در مرزهای خوشه همپوشانی داشته باشند، به درستی عمل نمیکند و برچسبهای سختگیرانهای را برای تعلق داشتن مشاهده به تنها یک خوشه اختصاص میدهد. بنابراین، نسخه جدیدی از مدلهای آمیزهای متناهی که با مفهوم تابع باور ادغام شده است، پیشنهاد می گردد. این مدل جدید راهکاری برای ایجاد بینش عمیقتر و احتمال تعلق داشتن مشاهده به فراخوشهها ارائه میکند. با در دست داشتن تابع درستنمایی، برخواسته از مدل آمیزهای نرمال، استفاده از روش براورد درستنمایی ممکن خواهد بود. اما در مدل آمیزه متناهی نرمال غالبا حل مسئله براورد به جوابهای بسته و واضح منتهی نمیشود. بنابراین پارامترهای مدل شواهدی با استفاده از یک الگوریتم بیشینهسازی امیدریاضی براورد و تعداد خوشهها با اندازهگیری معیار منحصربهفرد تعیین میشوند. عملکرد و مزیت این روش با استفاده از چندین مجموعه داده واقعی و شبیهسازی شده در مقایسه با چندین روش دیگر خوشهبندی مبتنی بر مدل و نمونه اولیه مورد تحلیل قرار گرفته است. در نهایت الگوریتم شواهدی عملکرد بهتری با در نظر گرفتن دقت و زمان داشته است.
- Abstract
-
When the homogeneity property of observations is easily violated in bigdata and high-dimensional data, data clustering is very important during an unsupervised learning process. Assigning the same label to data that is very similar to each other and very different from other data is a clustering process. One of the most useful clustering methods is when we use a finite mixture of statistical distributions, and the most common of them is a finite normal mixture. Previously, the performance of the normal distribution in clustering has been investigated, but the use of this distribution does not work properly when the observations overlap at the cluster boundaries and assigns hard labels to the observation as belonging to only one cluster. Therefore, a new version of finite mixture models integrated with the concept of belief function is proposed. This new model provides a way to gain deeper insight into the probability that observations belong to hyperclusters. With the likelihood function in hand, based on the normal mixture model, it will be possible to use the likelihood estimation method. But in the normal finite mixture model, solving the estimation problem often does not lead to closed and clear answers. Therefore, the parameters of the evidentical model are estimated by using a expectation maximization algorithm and the number of clusters is determined by measuring the unique criterion. The performance and advantage of this method have been analyzed using several real and simulated data sets compared to several other model-based and prototype-based clustering methods. Finally, the evidentiary algorithm has performed better considering accuracy and time.